Exploring the Dynamics of Quantitative Funds
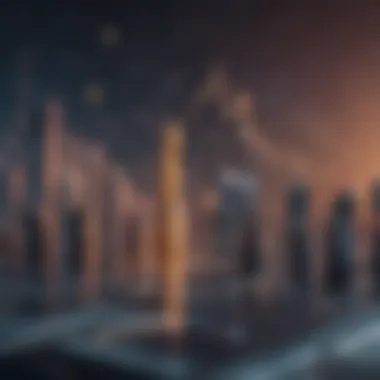
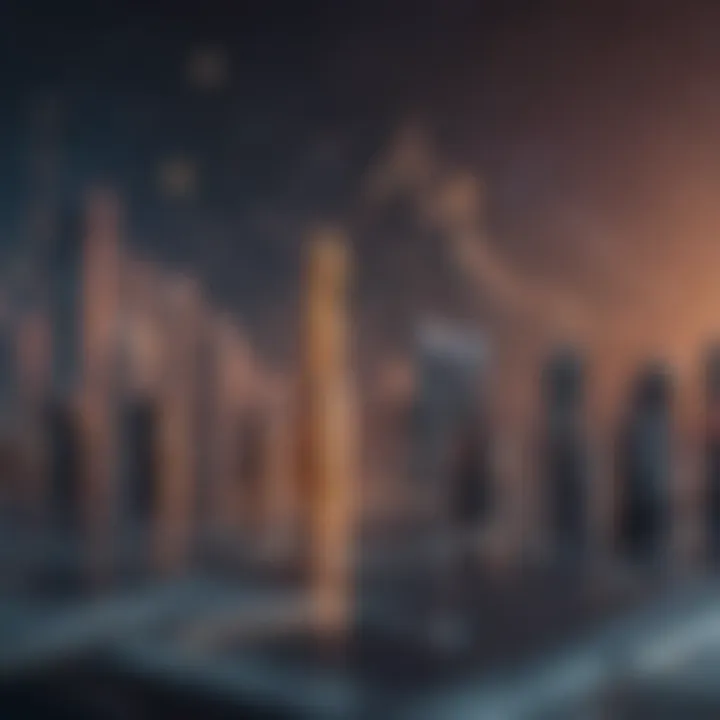
Intro
In todayās fast-paced financial world, understanding the mechanisms behind quantitative funds can provide investors with a competitive edge. As the landscape continues its rapid evolution, the structures and methodologies that define quantitative investing are more relevant than ever. Through this analysis, we seek to uncover the intricacies of these funds, peeling back layers to highlight operational techniques, challenges faced, and the potential horizon of quantitative strategies.
Quantitative funds utilize mathematical models and algorithms in their investment decision-making processes. The fusion of advanced statistics with high-speed data processing creates unique strategies that can operate far beyond human capabilities. Not only do these funds seek to identify patterns and trends, but they adopt a systematic approach to capturing opportunities across various markets. In light of this, a solid grasp of relevant terminology in quantitative finance becomes essential for both budding investors and seasoned professionals alike.
Preface to Quantitative Funds
When one brews a pot of coffee in the morning, the choice of beans, the grind size, and the infusion time play a pivotal role in determining the taste. Likewise, in the world of finance, the methodologies underpinning quantitative funds are intricate and foundational to how various investment strategies are crafted and executed. Understanding this realm is essential, as these funds are increasingly becoming a crucial part of the investment landscape.
Quantitative funds employ a data-driven approach to manage assets. They rely heavily on mathematical models and algorithms to make investment decisions, distinguishing themselves from traditional funds that may lean more on human intuition and discretion. Investors, financial professionals, and analysts must grasp these quantitative techniques, as they offer unique insights into market behaviors and the potential for achieving significant returns.
Furthermore, the advantages of utilizing such data-centric strategies are compelling. They not only aim for efficiency through automated processes but also strive for precision, focusing on vast data sets to uncover patterns that can lead to profitable trades. However, as with anything in finance, there are layers of complexity to navigate. The exploration of quantitative funds reveals both the opportunities and challenges present in modern investing.
Key Elements to Explore
- The definition of quantitative funds and their core principles.
- An in-depth look at the historical context giving rise to these funds and their growing prominence in the investment sector.
- The various operational aspectsādata collection, algorithm development, and risk management strategies vital in the functioning of quantitative investment models.
- Real-world examples and scenarios that illustrate the effectiveness of quantitative strategies over traditional methods.
Taking a step back to understand these factors will not just unearth the strengths of quantitative funds; it will also shed light on inherent challenges they face. For anyone involved in finance today, comprehending how quantitative funds operate and evolve is not just advantageous; it is indispensable for remaining competitive in a fast-paced market.
"In finance, as in life, knowledge is power, and those who embrace data will dictate the pace of the future."
By delving into the historical context and providing a clear definition of these funds, this article seeks to offer a compelling narrative that empowers readers to appreciate the intricate dance between numbers and decisions in quantitative investing.
The Mechanics of Quantitative Investing
The mechanics behind quantitative investing form the backbone of how these funds operate, influencing everything from data collection patterns to execution tactics. At its core, quantitative investing is about using mathematical models and algorithms to guide investment decisions. These methods stand in stark contrast to traditional investment approaches that often rely heavily on qualitative judgments and subjective interpretations.
A detailed understanding of the mechanics is pivotal for investors, financial advisers, and analysts who seek to grasp the sophistication embedded within quantitative strategies. The importance of this topic lies not only in mastering the quantitative techniques themselves but also in appreciating the complexities and potential pitfalls that can arise along the way.
Data Collection and Analysis
To successfully navigate the quantitative landscape, one must first grasp the process of data collection and analysis. Data is the lifeblood of quantitative investing; without high-quality, relevant data, models can falter. The data collection phase involves gathering vast quantities of information, which can include market data, economic indicators, and even social media sentiment.
Here's where it gets a bit tricky. Data isnāt just piles of numbers; it requires curation. For instance, a quantitative analyst may strip down stock prices to their raw data form, eliminating anomalies that could skew results. The relevance, reliability, and timeliness of the data are non-negotiable elements in this phase. Analysts need to be particularly cautious about data biases that might misguide the model outputs.
Data is typically processed through various algorithms that assess patterns over time, providing insights that inform the next steps of strategy formulation. If well executed, this phase can reveal trends that would escape a more traditional analyst's eyeālike spotting a sleeping tiger that is just about to awaken.
Algorithm Development
Once the data is meticulously gathered and scrutinized, the next step is algorithm development. In its simplest sense, an algorithm is a set of rules or processes designed to solve a specific problem. Quantitative funds leverage algorithms to identify investment opportunities based on the data theyāve unearthed.
This phase is where creativity meets technical proficiency. Itās not merely about crunching numbers; itās about crafting a framework that not only respects market complexities but also adapts to various economic scenarios. Successful algorithm development can be akin to teaching a child to ride a bicycleāthereās a mixture of guidance and freedom that needs to be established. An algorithm must be robust enough to follow, yet flexible enough to adjust.
Ultimately, a well-designed algorithm allows funds to engage in automatic trading, minimizing human interference and potentially enhancing efficiency. However, this approach isnāt foolproof; if the underlying data is flawed, the resulting trading strategies can miss their marks.
Risk Management Strategies
Risk management cannot be brushed aside, especially in the world of quantitative funds, where data storms can arise unexpectedly. At the heart of risk management is the recognition that all models are inherently imperfect. These models may perform brilliantly under certain market conditions but might flounder during periods of volatility.
Quantitative funds employ various risk management strategies to safeguard investments. These methods often involve scenario analysis, stress testing, and devising buffer zones to tolerate market fluctuations. Instead of simply reacting to market shifts, smart strategies incorporate predictive models designed to foresee potential downturns, balancing the portfolio against losses.
For instance, employing a stop-loss strategy can serve as a safety net for automated tradesāif an investment dips below a certain threshold, the algorithm will automatically sell the asset. This can help in minimizing losses, ensuring that funds cling to their capital even when the market seems like a rickety bridge.
In summary, the mechanics of quantitative investing hinge on the effective integration of data collection, algorithm development, and risk management. By comprehensively understanding these mechanics, investors and analysts can better appreciate the potent tools that quantitative funds wield in todayās increasingly complex financial ecosystem.
Types of Quantitative Funds
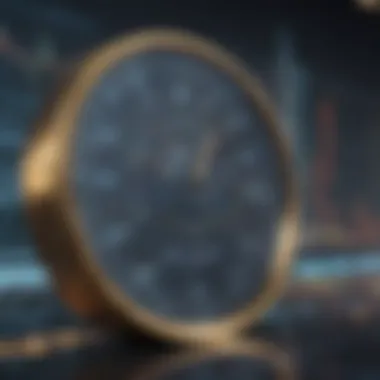
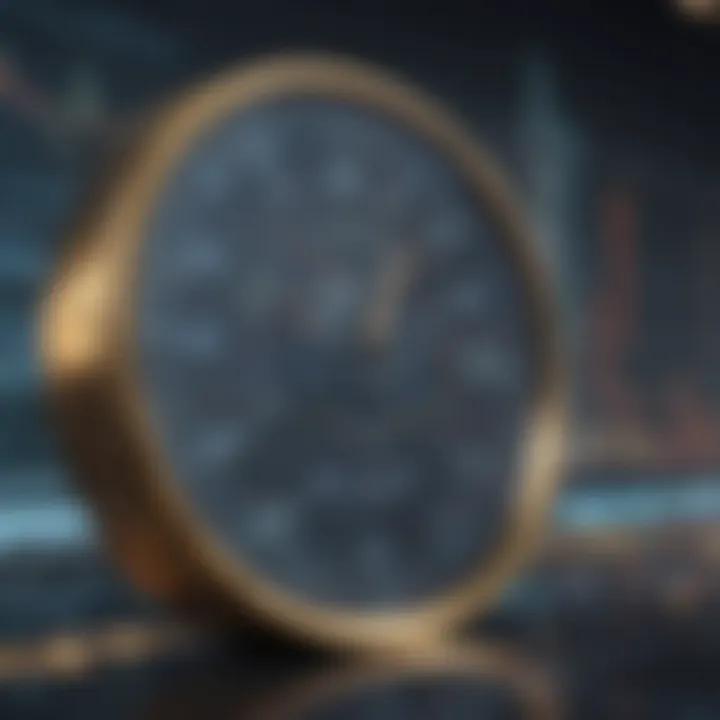
Quantitative funds are not just a monolithic category; they encompass various strategies tailored to distinct market conditions and investment goals. Understanding the types of quantitative funds is essential for investors and financial advisors as it provides a clearer picture of how these funds operate and the unique benefits each type offers. Each category has specific objectives, risk profiles, and methodologies that can help in portfolio diversification and optimizing returns. Here, we will explore three prominent types of quantitative funds: Market Neutral, Statistical Arbitrage, and High-Frequency Trading funds.
Market Neutral Funds
Market neutral funds aim to mitigate market risk by balancing long and short positions within a portfolio. The idea is that while the broader market may fluctuate, these funds can capitalize on the relative performance of the securities they hold. By maintaining equal dollar amounts in long and short positions, the impact of market movements is reduced, effectively neutralizing directional risk. This strategy can be particularly appealing in volatile or bear markets when traditional investment strategies may falter. Moreover, market-neutral funds often rely on rigorous statistical models and historical data analysis to identify mispricings between correlated assets, enhancing the potential for profit.
"The beauty of market neutral investing lies in its ability to generate returns irrespective of market direction, a piece of mind for risk-averse investors."
Key considerations for investors include:
- Volatility Control: Market neutral funds provide a strategy where the volatility of the portfolio can potentially be managed more effectively.
- Diversification: They can offer a diversification benefit in a portfolio that includes traditional long-only funds.
- Skill-Dependent: Success largely hinges on the fund manager's ability to create and maintain a balanced portfolio.
Statistical Arbitrage Funds
Statistical arbitrage funds employ sophisticated algorithms and statistical methods to identify mispricings among related financial instruments, often relying on pairs trading strategies. The essence of these funds lies in exploiting statistical anomalies, or mean reversion, where prices deviate from their historical norms. This type of fund operates on the premise that deviations are temporary, and prices will eventually revert, creating a profitable trade opportunity. Analysts dive deep into complex data sets to make predictions, sometimes making hundreds of trades in a single day.
Investors looking to understand statistical arbitrage should note:
- Rapid Execution: These funds typically execute trades swiftly, often relying on quantitative models to analyze factors like price movements and volume.
- High Leverage Use: They often apply leverage to magnify gains but bear in mind it can amplify losses too.
- Limited Market Exposure: The goal is to capture small profits from numerous transactions rather than depending on market trends.
High-Frequency Trading Funds
High-frequency trading funds represent one of the most technologically advanced approaches in the realm of quantitative investing. These funds leverage advanced algorithms and cutting-edge technology to execute a large number of trades at extremely high speeds. The goal is not just profit from typical market movements but to profit from imbalances and inefficiencies that exist for mere seconds or milliseconds. As such, high-frequency strategies often involve short time horizons and very small profit margins per trade.
For investors and professionals looking into high-frequency trading, several aspects stand out:
- Infrastructure Investment: Successful high-frequency trading requires substantial investment in technology and infrastructure to minimize latency and maximize execution speeds.
- Data Analytics: The reliance on real-time data and analysis cannot be overstated; successful trades are often predicated on timely information.
- Regulatory Scrutiny: Given the firm's rapid-fire trading nature, it often finds itself within the purview of regulators who are concerned about market fairness and transparency.
Understanding the various types of quantitative funds empowers investors to align their strategies with the right fund depending on their risk tolerance, desired involvement level, and overall market outlook. As we move further in the article, we will explore the advantages these funds offer and delve into potential challenges associated with them.
Advantages of Quantitative Funds
Quantitative funds have become a significant player in the investment world. Understanding their advantages gives investors a clearer picture of why many are shifting towards data-centric investment approaches. How these funds leverage statistical techniques can pave the way for smarter financial decisions. Hereās a closer look at the specific benefits that set quantitative funds apart.
Data-Driven Decision Making
One of the most compelling advantages of quantitative funds lies in their reliance on data-driven decision making. This approach minimizes human biases, which can often cloud judgment in volatile markets. Instead of relying on gut feelings or market rumors, quantitative funds analyze vast amounts of data ranging from historical prices to economic indicators.
For instance, when considering a stockās potential, a quantitative fund will use algorithms to assess patterns that the average investor might miss. These insights inform tradesānot through emotions or trends but rather through cold, hard facts. By employing rigorous statistical models, these funds can identify opportunities where traditional investing might falter. Here, numbers speak louder than opinions, leading to potentially higher returns.
Consistency in Strategy Execution
Consistency could be considered the holy grail of investing, and quantitative funds offer a level of strategy execution that is hard to match. This consistency stems from automated processes that follow clearly defined rules. Once algorithms are set up to analyze data and make trades, they stick to their guns without deviating from their programmed logic.
This approach is beneficial, especially in times of market turbulence. While other investors may panic and react impulsively, quantitative funds remain steady, executing trades according to the strategy. This kind of reliability helps to maintain performance across various market cycles. Just think of it this way: in a stormy sea of financial markets, quantitative funds are like a well-calibrated shipāsteady and true, even as waves crash all around.
Diversification Benefits
Diversification is another important advantage of quantitative funds. By applying sophisticated mathematical models and analytics, these funds can assess and construct portfolios that spread risk across multiple asset classes. Rather than picking a handful of stocks or bonds, they can analyze hundreds or even thousands of investment opportunities simultaneously.
This widespread analysis leads to a portfolio that is not only well-diversified but also tailored to the specific risk preferences of investors. For example, a quantitative fund may focus on optimizing asset allocation between various sectors, geographies, or even types of investment. In doing so, it reduces the likelihood of encountering significant losses during market downturns, while simultaneously capturing potential gains across different areas of the market.
"Quantitative funds leverage technology and data analytics to outperform traditional investing, offering consistent execution and diversification."
By understanding these key advantages, investors can appreciate why quantitative funds may present a compelling alternative to more traditional investment strategies. They illustrate how blending advanced technology with financial acumen can result in better decision-making, enhanced consistency, and a more diversified approach to risk and opportunity.
Challenges Faced by Quantitative Funds
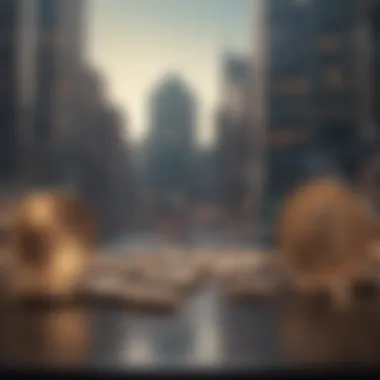
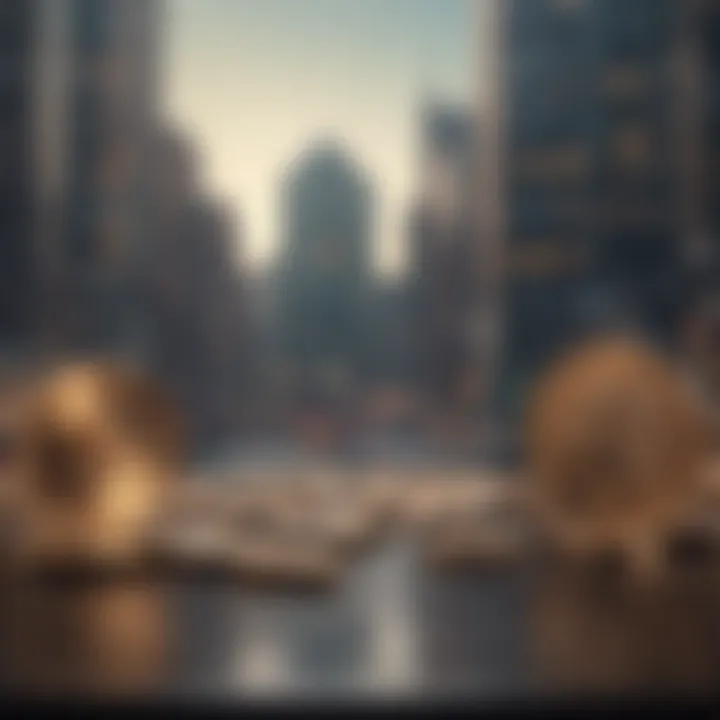
In the realm of quantitative investing, challenges aren't just bumps in the road; they can be formidable obstacles that may derail even the most sophisticated strategies. Understanding these challenges is vital for investors and financial professionals alike. Quantitative funds thrive on data and algorithms, but those very foundations can lead to pitfalls, often overlooked by the untrained eye. Addressing the potential challenges ensures that these funds can adapt, thrive, and continue to deliver value in an ever-evolving market landscape.
Model Overfitting
Model overfitting is akin to a tailor crafting a suit so precisely fitting that it's no longer wearable. In essence, when a model is trained too closely to the historical data, it captures noise in the dataset rather than the actual underlying trends. This leads to poor predictive performance on new, unseen data.
One way to illustrate this is by considering two different analytics models: one that predicts stock performance based on the last two decades of data and another that uses a more general approach taking broader market indicators into account. The first model may seem to provide impressive results; however, when market conditions shift, its predictions misfire. Investors need to be wary of strategies that seem too good to be true, as they might be resting on shaky foundations.
To counteract these effects, analysts often employ techniques like cross-validation or regularization. By rigorously testing models against various data subsets, they can discern between genuine signals and mere coincidences.
Market Regime Changes
Just like seasons change, market regimes shift too. Understanding the current regime ā be it bull market, bear market, or some other phase ā is crucial for quantitative funds. If a model assumes the prevailing trends will persist indefinitely, it may fall flat when the winds of change blow. These regime changes can be sparked by economic indicators, geopolitical events, or shifts in market sentiment, all of which can substantially impact performance.
Investors must be prepared for this unpredictability. A model that performed brilliantly in a sustaining bull market may face challenges when sentiments turn sour. Diversification across various assets and adapting models in real-time can help cushion the blow of sudden market changes. A keen awareness and quick adjustment are fundamental to sustaining value in the face of fluctuating market regimes.
Data Quality Issues
Data isnāt simply numbers on a page; itās the lifeblood of quantitative funds. The saying, "garbage in, garbage out" rings especially true here. Data quality issues can undermine strategies and lead to misguided investment decisions. If the data being fed into algorithms is flawedābe it through inaccuracies, biases, or incompletenessāthe output will inevitably reflect those deficiencies.
Consider a scenario where a fund relies on market sentiment data harvested from social media. If the algorithms donāt accurately parse sentimentāa sentiment fueled by bots or manipulated trendsāthe fund could misinterpret market indicators, leading to investment missteps.
To mitigate these risks, thorough vetting and continual monitoring of data sources is essential. This could involve employing advanced cleansing techniques or leveraging technology like blockchain for data integrity. Only by ensuring high-quality inputs can the algorithms produce reliable outputs.
Technological Impact on Quantitative Funds
The intersection of technology and quantitative investing is not just a small chapter in the story; itās the core around which the narrative revolves. Technological advancements have not only changed the way that quantitative funds operate, but they have also broadened the capabilities of such funds to leverage data, adapt to market shifts, and optimize strategies in real-time. These developments have made for an ever-evolving landscape that presents exciting opportunities yet also nuanced challenges.
Artificial Intelligence and Machine Learning
Artificial Intelligence (AI) and Machine Learning (ML) stand at the forefront of technological innovation within quantitative funds. These technologies enable systems to learn from data patterns without explicit programming. In an environment that's as fluid and chaotic as financial markets, AI can sift through vast amounts of dataāa task that would take humans far longerāto spot trends and generate actionable insights.
- Predictive Modeling: By applying machine learning algorithms, funds can create models that predict price movements based on historical data, enabling them to make informed investment decisions.
- Sentiment Analysis: AI isn't limited to numerical data alone. By analyzing social media, news articles, and other textual data, funds can gauge market sentiment and adjust strategies accordingly.
With everything working in harmony, portfolio management becomes more dynamic. As one might say, using AI in investing is like having a hungry wolf skillfully tracking its prey through the woods. The computational prowess helps identify lucrative opportunities while managing risks effectively.
Big Data Analytics
The term "Big Data" might sound like corporate jargon, but in the context of quantitative funds, it means transforming mountains of information into usable intelligence. Traditional financial metrics and historical data can only scratch the surface; big data dives deeper into non-traditional data setsāsocial trends, weather patterns, and even satellite imageryāto give analysts a holistic view of potential investments.
- Enhancing Decision-Making: With access to vast amounts of information, metrics can be re-evaluated, allowing firms to make timely, data-informed decisions.
- Risk Assessment: Big Data provides the ability to analyze risk in a comprehensive manner, identifying hidden correlations and potential threats before they become significant.
Such analytical depth means that quantitative funds can pivot quickly and effectively. Adapting strategies based on real-time data feeds is akin to adjusting sails on a ship; it keeps the vessel steady even amidst turbulent seas.
Blockchain and Its Implications
Blockchain technology has rapidly gained attention across various sectors, and its implications for quantitative funds cannot be ignored. This decentralized ledger technology offers transparency, traceability, and a high degree of security, which is crucial in today's data-centric world.
- Transaction Efficiency: By enabling faster transactions and eliminating the need for intermediaries, blockchain reduces costs significantly. For quantitative funds, this means the ability to execute strategies swiftly and efficiently.
- Data Integrity: Ensuring that data has not been tampered with is vital for the accuracy of modeling. Blockchain provides a way to verify and authenticate data, fostering trust in the information that drives investment decisions.
To sum it up, whether it's AI enhancing predictive capabilities, big data refining decision-making processes, or blockchain ensuring data integrity, the impact of these technologies on quantitative funds is profound. They don't just complement traditional methods; they redefine the very fabric of how investing is approached today.
"Technological advancements aren't simply tools; they are the backbone upon which the future of quantitative investing rests."
In the landscape of finance, where variables shift like sand in the wind, leveraging these technologies allows quantitative funds to take full advantage of market inefficiencies, potentially driving greater returns for investors.
Performance Measurement
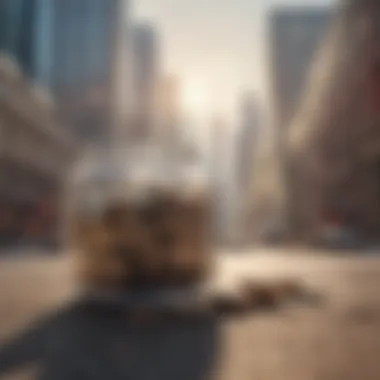
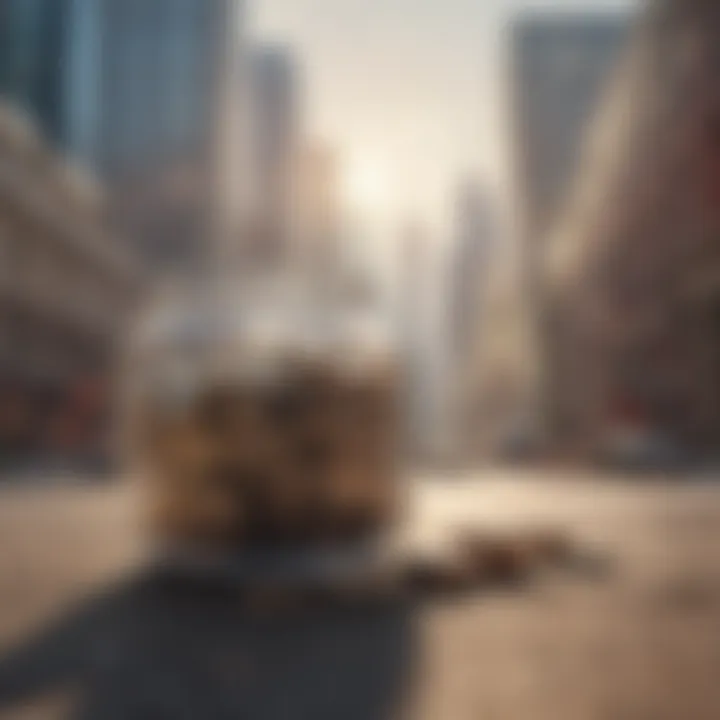
Performance measurement serves as the backbone of understanding how quantitative funds deliver results. Unlike traditional investing, where qualitative factors often play a significant role, quantitative investing hinges on objective metrics. This is critical because it enables fund managers and investors to discern which strategies are working and which aren't.
To gauge success accurately, practitioners lean on a set of established and emerging criteria. Some of the primary benefits of performance measurement in quantitative funds include the following:
- Transparency: Investors benefit when managers apply a systematic approach to assess performance. This transparency fosters trust and can lead to lasting partnerships.
- Informed Decisions: By evaluating metrics regularly, fund managers can pivot quickly if specific strategies underperform. This adaptability is key in the ever-shifting financial landscape.
- Goal Alignment: Understanding performance helps ensure that the fundās objectives align with investor expectations.
Key Performance Indicators
Key performance indicators (KPIs) are quantifiable metrics that monitor the fund's success. They serve as the yardstick for performance, offering insights ranging from risk-adjusted returns to volatility. Some of the pivotal KPIs that quantitative funds might track include:
- Sharpe Ratio: This ratio indicates how much excess return is received for the extra volatility endured by holding a riskier asset compared to a risk-free asset. Higher Sharpe ratios suggest more attractive risk-adjusted returns.
- Alpha: A measure of performance that compares the return of the fund to a benchmark index. A positive alpha shows that the fund has outperformed the index, while a negative number suggests underperformance.
- Sortino Ratio: Similar to the Sharpe Ratio, but it focuses on downside risk only, making it a better indicator of performance in negatively trending markets.
These indicators, while vital, should not be interpreted in isolation. Their effectiveness improves when used in conjunction with one another, creating a composite view of performance.
Benchmarking Against Traditional Funds
Benchmarking provides a frame of reference. When quantitative funds are measured against traditional funds, investors can gauge whether the quantitative approach holds its own.
- Comparative Analysis: It helps in assessing performance in relation to established benchmarks such as indexes or peer funds. This context adds depth to the evaluation, helping identify trends and performance anomalies.
- Risk-Adjusted Returns: When comparing a quantitative fund to traditional counterparts, performance metrics must account for the different risk profiles each type of fund sports. Understanding how these elements intertwine gives a clearer picture.
- Adaptability to Market Conditions: A set benchmark allows one to see how a quantitative fund fares in various market conditions compared to traditional strategies. If a fund consistently outperforms during turbulent times, it signifies robust risk management and forethought.
Conclusively, the ongoing assessment of performance is not merely a box-ticking exercise; it is central to maintaining the integrity and longevity of a quantitative fund. By trusting in a data-centric approach, both fund managers and investors can stay a step ahead in today's intricate market environment.
Future Trends in Quantitative Investing
The landscape of quantitative investing is constantly shifting, driven by advancements in technology and changing market dynamics. Understanding the future trends in this field is not just intriguing for finance enthusiasts but essential for investors who want to stay ahead of the curve. These trends can reshape how quantitative funds operate, influencing their strategies and risk frameworks. As we delve deeper, we will explore three significant trends: the integration of ESG factors, the rise of retail quant funds, and the evolving regulatory landscape.
Integration of ESG Factors
In recent years, Environmental, Social, and Governance (ESG) factors have garnered significant attention within the investment community. Traditionally, quantitative funds focused solely on financial metrics and market data. However, as public awareness and concern for sustainability heightened, thereās increasing pressure on these funds to incorporate ESG criteria into their investment models.
Quantitative funds can leverage sophisticated algorithms to assess ESG data alongside traditional financial metrics. This integration does not only align with the growing demand for socially responsible investing, but it also enhances the overall risk profile of the portfolio. Considering factors such as carbon footprint or corporate governance can lead to more informed investment decisions, ultimately yielding better long-term returns. Integrating ESG factors is not just a trend; itās becoming a necessity for funds that aim to attract a wider investor base.
Rise of Retail Quant Funds
The barriers to entry for quantitative investing have significantly lowered in recent years, thanks to advancements in technology and the democratization of financial data. Retail investors now have access to powerful tools that were once the domain of institutional investors. This shift is resulting in a surge of retail quant funds, where individual investors can apply quantitative strategies on personal portfolios.
These retail quant funds often utilize online platforms that provide ready-made algorithms or customizable models. Investors are searching for an edge in a volatile market, and quantitative strategies offer a way to automate the decision-making process based on data rather than emotions. As more retail investors dip their toes into quantitative investing, it will also be interesting to observe how these funds impact market dynamics, potentially leading to increased volatility due to the speed at which trades occur.
Evolving Regulatory Landscape
As quantitative investing continues to evolve, so does the accompanying regulatory environment. Regulators are becoming increasingly attuned to the complexities and potential risks associated with algorithmic trading and automated decision-making. As a result, compliance requirements are likely to change, affecting how quantitative funds can develop and deploy their strategies.
For instance, concerns about market manipulation and system stability may lead to stricter oversight of high-frequency trading practices. Funds will need to navigate these evolving regulations carefully to ensure they meet compliance while still reaping the benefits of advanced technologies. Furthermore, transparency in algorithms and data management could become a major focus for regulators, influencing how funds present their methodologies to investors.
"Today's quants are not just mathematicians or programmers; they are also emerging as advocates for thoughtful regulation that fosters innovation while safeguarding market integrity."
Overall, staying informed about future trends in quantitative investing is critical for stakeholders across the boardāfrom institutional investors to individual retail traders. The integration of ESG factors will align investment strategies with global priorities, retail quant funds will democratize access and implementable strategies, and the evolving regulatory landscape will demand agility and adaptability. These trends are set to redefine the playbook for quantitative funds in the coming years.
Closure
Summarizing Key Points
- Defining Characteristics: Quantitative funds leverage data and statistical methods to inform investment strategies. By employing algorithms, these funds strive to minimize human biases and enhance decision-making.
- Technological Advancements: The role of technology in quantitative investing cannot be overstated. Innovations in machine learning and big data analytics have transformed how data is collected and analyzed, allowing managers to uncover patterns and opportunities previously hidden from traditional approaches.
- Risk Management: The emphasis on quantitative risk management techniques is a significant benefit, ensuring that funds can navigate market volatility more effectively than many conventional methods.
- Future Considerations: The integration of ESG factors and the rise of retail quant funds signal considerable shifts in the investment landscape. Itās vital for investors to stay abreast of these trends, as they have direct implications on strategy and portfolio management.
By synthesizing these key elements, one can appreciate that quantitative funds offer a pragmatic approach to investment, grounded in data and reinforced by technology.
Final Thoughts on Quantitative Funds
Quantitative funds stand at a crossroads of tradition and innovation in the investment world. They embody a shift toward data-centric strategies, where superior mathematical models and computing capabilities dictate market movements rather than mere intuition. For investors and advisors, gaining clarity on this topic is indispensable. With the financial markets becoming more complex, quantitative investing presents an opportunity to navigate these waters with greater precision.
Investors need to remain vigilant about the challenges these funds face, such as model overfitting and data quality issues. Itās not a one-size-fits-all solution. By comprehensively understanding these funds and their operating landscapes, one can make informed investment choices that align with their financial goals.
"In the realm of finance, the more you know, the more empowered you become."
Itās essential to reflect on personal investment objectives, as quantitative funds are not just another vehicle; they represent a distinct philosophy of investing. As the market continues to evolve, so too will the strategies employed by quantitative funds. Staying informed is key for anyone looking to ride the wave of this investment strategy.